Generative AI: sustainability game changer or ticking time bomb?
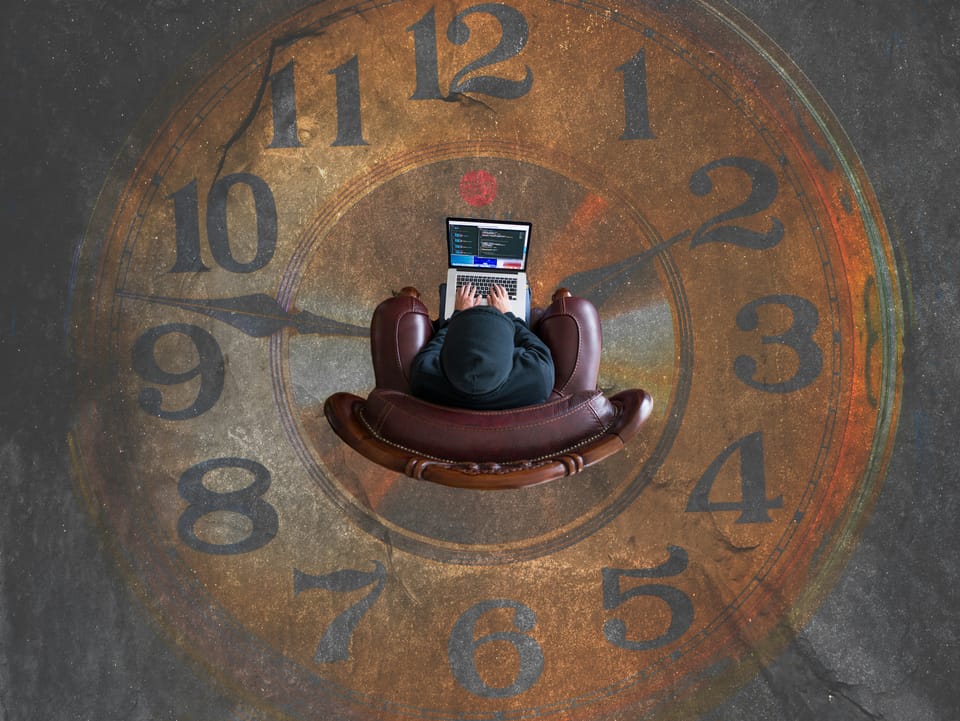
From creating tattoo designs to optimising energy systems, Artificial Intelligence (AI) is permeating almost every aspect of our lives – including the work of Chief Sustainability Officers, where it offers compelling capabilities.
For Chief Sustainability Officers, AI has the potential to be a game-changer. Not only can it help them track and optimise complex systems to reduce food, water or energy waste, and predict or detect risks such as wildfires or methane leaks – AI also has the power to act as what Microsoft Chief Sustainability Commercial Officer Sherif Tawfik described as “a copilot for sustainability professionals”.
“Imagine a Chief Sustainability Officer having a copilot that can engage thousands of suppliers, helping the suppliers and filling the survey, bringing those data, crunching the data, simplifying it, detecting anomalies. In all of those scenarios, AI can help with complex data gathering and analysis,” he told reporters on the sidelines of COP28 last December. (Tawfik, of course, has skin in the game here: Microsoft sells such Copilot-branded tools.)
AI for environmental impact mapping
Data (especially Scope 3 data) is the thorn in CSOs’ side, and this is where generative AI can make the biggest difference, experts in the space suggest.
Deepak Ramchandani-Vensi, Consulting Director at AI and data consultancy Mesh-AI, explains that AI is currently most powerful in helping companies get an accurate perspective of what their – and their suppliers’ – current sustainability metrics are.
“Organisations can leverage technology like large language models to process thousands of documents from across their supply chain to understand what their suppliers are doing around sustainability. They can use that data to look at areas to partner with them, or consider changing suppliers where they do not have a vision match,” he tells CSO Futures.
Data experts have previously warned that AI is only as good as the data it is fed, but some now think this problem is already being overcome: thanks to the sheer volume of data analysed, generative AI can help users identify inaccurate data and prompt them to look for better inputs.
“What the AI revolution has really shown is basically, more data, big data, beats smart algorithms. Put very, very simply, if you brute force it with enough data, then it comes up with really incredible answers and insights, as we know from ChatGPT,” says John Ridd, CEO of cloud emissions data firm Greenpixie. (Some data scientists say that so-called small language-models or those trained on proprietary data using a technique called Retrieval Augmented Generation may be more effective for certain tasks than large cloud-based models.)
Democratising sustainability action
The brilliance of generative AI is that it can “democratise action”, Ridd adds: where only an expert could previously understand and interpret these complex data sources, AI provides insights from the same sources in natural language, allowing just about anyone to act on them.
By taking over repetitive tasks and data gathering, AI is also freeing up sustainability professionals to focus more on strategy and innovation – a necessary shift to truly integrate sustainability into core business.
For example, Mesh-AI claims that it has helped large clients refine supply chain processes, finesse product design, optimise specific third-party production processes, and consider the potential benefits of reverse logistics and recycling initiatives.
Recently, Apple enabled ‘clean energy charging’ for iPhone users, allowing them to charge their phones during times of cleaner energy production on their local grids – effectively using AI to lower Scope 3 emissions.
Generative AI and its power needs
But there is a potentially massive downside to integrating AI into sustainability work. Generative AI applications are incredibly power-hungry to train and use – so much so that OpenAI CEO Sam Altman believes there is no way for AI to reach its full potential without new energy sources (the company is investing heavily in nuclear fusion).
The energy consumed to train the GPT-3 model is estimated around 1,064 MWh. Meta reportedly said last year that it consumed 2.6 million KWh of electricity and emitted 1,000 tonnes of CO2 to train its new LLaMA models – the equivalent of 254 gasoline-powered cars driven for a year.
To make AI ever more efficient, accurate and predictive, developers are feeding applications ever more data and parameters, increasing the energy consumption needed to process them. According to chip manufacturer Synopsis, a few years ago, it would take 27 KWh to train a model – today, it takes half a million KWh.
Between 2012 and 2018, the computing power required to train AI foundation models increased by a factor of 300,000 – roughly doubling every 3.4 months, according to OpenAI. As well as training, simply using AI is also incredibly energy-intensive: Alphabet's Chairman John Hennessy told Reuters last year that a query on a large language model like ChatGPT used 10 times more power than a standard keyword search.
AI’s environmental impacts should not be ignored
The reason for that is that generative AI applications run on computing engines called graphics processing units (GPUs), as opposed to central processing units (CPUs). GPUs deliver a much stronger performance than CPUs by running many different tasks in parallel, making them more energy-intensive.
Generative AI is not just power-hungry: it is also extremely thirsty: researchers estimate that training GPT‑3 alone in Microsoft’s US data centres consumed 700,000 litres of clean freshwater – and that does not include the off-site water footprint associated with electricity generation.
For Chief Sustainability Officers, these are crucial numbers to consider. “There’s been a lot of coverage on the social repercussions of AI, which can be huge, but the environmental side has barely been covered. And it really is a ticking time bomb,” says Ridd.
Mitigating the risks of using AI for sustainability
There are ways to make sure the negative impacts of AI do not jeopardise companies’ sustainability efforts, adds the Greenpixie CEO.
“There's a couple of decisions that can be made, and this can go into the sustainability repercussions of AI as well,” he says.
There are three potential ways to implement AI within organisations: having a subscription to a tool like ChatGPT and using it on a browser; building your own closed AI application using enterprise cloud; or doing the same on premises.
The first option, simply using the public version of an AI tool, means using public data – and making any data shared with the algorithm public.
“You could also have a copy of ChatGPT or equivalent within your own environment: if you're a large enterprise, you could then hook in all of the information that you have. If you were to do that on the public version of ChatGPT, that's gonna be a big security risk,” says Ridd.
Not only that, but running an AI model on an internal IT system also dramatically increases visibility and control over environmental impacts. AI companies are largely opaque when it comes to the emissions of their products.
OpenAI has disclosed no information about its impact on its website.
One staff member answered a developer question on the topic by saying it’s “trending downwards towards zero”, since its infrastructure runs on Azure, which aims to run on 100% renewable energy by 2025, but admitted that “we haven’t done that full calculation”.
Relying on cloud providers’ 100% renewable energy claims is problematic for any cloud user, since these are mostly based on market instruments like renewable energy certificates, with most data centres still running on dirty grids.
But using an AI model hosted on an enterprise cloud makes it moderately easier to track this impact, either by requesting specific data centre information from cloud providers, or by contracting IT emissions tracking software.
Efficiency and transparency on the mind
“The second you go towards enterprise, you're in all likelihood using AI more intensively, and you are then definitely responsible for those emissions under your Scope 3 at that point,” he warns.
But that also means being able to choose how to use AI to limit negative impacts, for example by using data centres in locations with cleaner grids , or encouraging employees to use it only when necessary, prioritising task-based computation over GPUs wherever possible.
“Lastly, if you're training an LLM or even building your own, choosing the location and time at which you are doing this computation will make a big difference. So if you do it at certain times of the day, when there's low carbon intensity or at low-carbon location, then you can minimise your carbon footprint,” advises Ridd.
The use of AI by Chief Sustainability Officers, in short, is one of trade-offs. There are potentially significant “micro” efficiencies to be generated by putting the power of AI to work on enterprise data, including around minimising an organisation’s carbon footprint.
But without more transparency from providers on emissions and water use – and a deeper conversation about the growing pressure AI-centric data centres are putting on already stressed electricity grids, the “macro” consequences of AI could do sustained damage. More work on impact clearly needs to happen.
Member discussion