Addressing climate change with data: the good, the bad, and the (un)certain
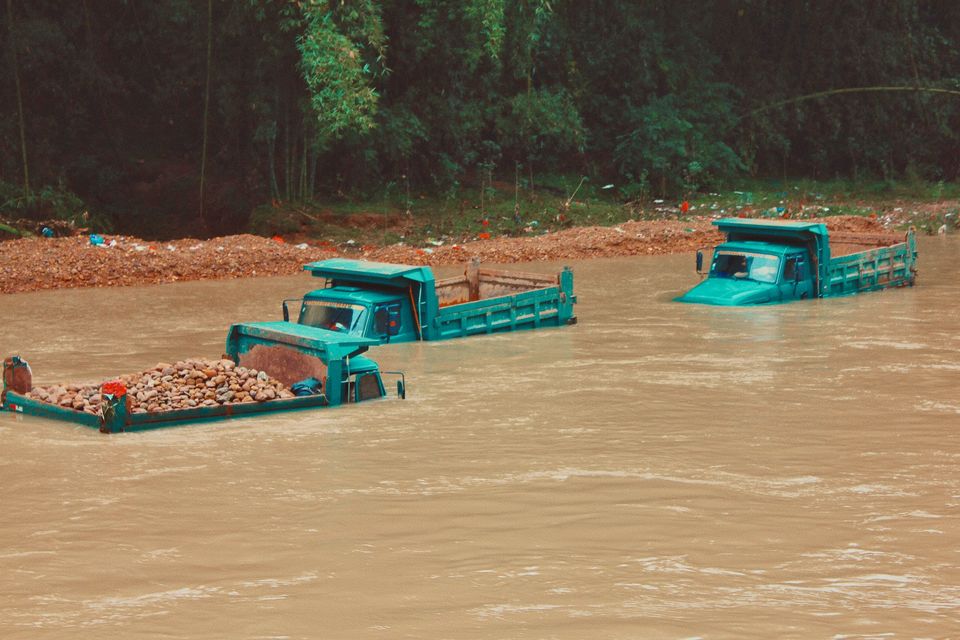
Climate change is here to stay but there is, by now, plenty of data available which can be used to address both the physical and transition risks associated with it, write Dr Jannis Hoch, senior hydrologist and Conor Lamb, catastrophe model developer at climate risk specialist Fathom.
These data have the potential to tell us a lot and must be central in our approaches to tackling climate change. However, the production, processing and usage of such data involves a multitude of limitations and uncertainties that must be considered for effective and informed decision making. Within this context, the communication of uncertainties, limitations and modeling decisions must become as valued as the data itself. It is only then that these data can be used at their full potential.
When it comes to addressing physical risks due to climate change, let’s first establish where new data stems from:
- Firstly, earth observation data, which is predominantly produced by satellite missions and yields globally consistent measurements of many natural variables.
- The second major data source is the outputs from general circulation models. These are computational attempts to model the Earth's atmosphere by solving fundamental physical equations. Circulation models at a global scale tend to be run at a coarse resolution due to the huge computational requirements.
- Third, we have ground observations of natural variables such as temperature, rainfall or river flow.
- Finally, impact models use the data from the above to estimate the impacts of climate change on natural hazards such as drought, wildfire, flooding and tropical cyclones, as well as their interactions with humans and the built environment.
These data sources and modeling approaches have allowed us to obtain an unprecedented understanding of the processes at play whilst the climate changes. However, each is subject to a multitude of inherent uncertainties and limitations, which often multiply as the data is repeatedly processed before being supplied to end-users. Furthermore, the uncertainties of model inputs are often not fully propagated throughout the modeling chain, resulting in poor estimates of model output uncertainty available to the end user.
Take, for instance, the current generation of general circulation models. The underlying processes have been researched for decades and are now understood well enough to make many evidenced predictions about the climate of tomorrow. However, these models are designed for evaluating long term trends and large scale patterns. As such, models often struggle with representing rare or extreme events that risk professionals are interested in. Furthermore, the work of converting these trends and patterns to risk models is convoluted and the uncertainty is rarely represented throughout.
We are also limited by the availability of data for validation and calibration; typically ground observations and earth observation data. Here, the rare nature of extreme events means that data for calibrating models is scarce. Similarly, hazard models are calibrated in areas where there are available historical records; often in more economically developed countries. As a result, despite using the same methods globally, the accuracy of the model output is likely to decrease in areas without suitable historical records, resulting in inequitable data quality.
This issue is often exacerbated by techniques such as artificial intelligence (AI). These approaches, whilst exceedingly powerful when used appropriately, may be used to cover poor data quality or a lack of physical representation of climate systems; instead substituting in highly precise models with little indication of model skill. The growing number of vendors selling AI-based data should be treated with a healthy skepticism. Especially where these vendors claim “certainty” without acknowledging “uncertainty”.
See also: Sustainability-linked loans are not (yet) where they need to be
In order for new data to be used in an appropriate manner, we must ensure that the uncertainty in model inputs are fully propagated through the modeling chain and reported to the end user. Only then is the true uncertainty (including the compounding effects of inequitable data quality) fully realized and can be accounted for.
However, decision makers, including risk managers and businesses looking to use such data, must also adapt to take their uncertainty into consideration. In particular, decision making tools and impact assessments must evolve to explicitly consider the uncertainties arising for variable quality inputs and the compound effects of the modeling chain.
A good example of how to deal with uncertainties exists within the insurance industry and its use of catastrophe models. These models assess the risks to an insurer’s portfolio and return not just an assessment of the average yearly risk, but also the tail risks. Tail risks refer to the impacts within a bad year.
For example, insurers will often use a loss which represents their worst year from a set of 200 years (providing a 0.5% chance of exceeding this loss in any given year), to evaluate how much money they need to save in order to pay out claims in an exceptionally bad year. Whilst this example looks to uncertainty arising from a highly variable system (yearly insurance losses from natural disasters), the lessons learnt can readily be transferred to climate change risk and adaptation. Namely, risk managers should model and plan for the reasonable worst case scenarios arising from the suite of climate data they utilize, as opposed to a median outcome. Furthermore, subjective decisions (in this example the 200 year threshold) should be transparently stated and open to scrutiny. These steps lead to more robust decision making and more resilient systems.
To conclude, the data for addressing climate change have never been more prevalent and will be vital in minimizing the impacts. However, whilst continuing to ensure greater data availability, especially in currently data-scarce areas, we should focus on reducing data misuse and ensuring the ability to process such data while being aware of inherent uncertainties. We should also look to develop models which quantify all uncertainty wherever it arises, and pass estimates to end users such as decision makers. To that end, uncertainty estimates should not be considered obstructive. Instead, risk managers and other data users must become comfortable living within this uncertainty and take steps to prepare for the entire range of possible scenarios and impacts climate change may bring. It is only then that unwanted surprises can be avoided and risk managers can utilize the full potential of climate risk data.
Member discussion